Learning Analytics and Adaptive Learning Focused on Mathematics
Adaptive learning means a learning system that continuously adapts itself to the abilities and development of the learners. For mathematics this can mean that learners are always given the appropriate math task in which they can apply their previous knowledge and at the same time acquire the knowledge needed for the next task. AI provides the algorithms needed to do this.
Digital exercises were developed for the learning management systems Moodle and ILIAS, which new students can use to refresh their knowledge from school. The exercises have now been tested with around 800 students at four universities in North Rhine-Westphalia and Hesse (as of spring 2024). The associated learning data is constantly being analyzed anonymously in order to derive scientific findings and improve the exercises.
First and foremost, gamification elements support learning. Three different designs – standard, pedagogical agent and fantasy game design – offer students learning materials to suit their preferences. Collecting and analyzing the learning data shows that the different designs have different effects on learning behavior. For example, exercises in the fantasy game design are practiced much more frequently due to students repeating them.
In addition, the tasks are equipped with an interface for adaptive learning. As an example from the pedagogical agent design: If learners are unable to solve the most difficult exercise in one topic, they are first directed to an overview of that topic, showing their current learning progress, so that they can select the task that suits them best.
A survey among students in the mathematics preparatory courses of the winter semester 2022-23 shows that students experienced an increase in enjoyment from the exercises.
The exercises are made available open-source, intended for replication and further development. The Moodle course (Moodle course export - MBZ file), as well as the code are freely available for interested parties (MIT license): To the GitLab repository.
The exercises can be tried out at anytime in an open Moodle instance: To the open Moodle course.
The whole learning solution can also be made available in your own learning management system, either by copying the course files from the repository or via an e-learning community server.
Publications
- Neugebauer, M., Erlebach, R., Kaufmann, C., Mohr, J.,Frochte, J. (2024). Efficient Learning Processes by Design: Analysis of Usage Patterns in Differently Designed Digital Self-Learning Environments. In: Proceedings of the 16th International Conference on Computer Supported Education (CSEDU 2024) - Volume 2. Pages 476-477. DOI, PDF, BibTex
- Neugebauer, M. (2024). Lightweight Learning Analytics Dashboard for Analyzing the Impact of Feedback & Design on Learning in Mathematical E-Learning. In: Proceedings 18. Workshop Mathematik in ingenieurwissenschaftlichen Studiengängen. Pages 44-49. PDF, BibTex
- Neugebauer, M., Tousside, B.,Frochte, J. (2023). Success Factors for Mathematical E-Learning Exercises Focusing First-Year Students. In: Proceedings of the 15th International Conference on Computer Supported Education (CSEDU 2023) - Volume 2. Pages 306-317. DOI, PDF, BibTex
- Neugebauer, M.,Frochte, J. (2023). Steigerung von Lernerfolg und Motivation durch gamifizierte Mathematik-Aufgaben in Lernmanagementsystemen. In: Proceedings of the 21. Fachtagung Bildungstechnologien (DELFI 2023). Pages 247-248. DOI, PDF, BibTex
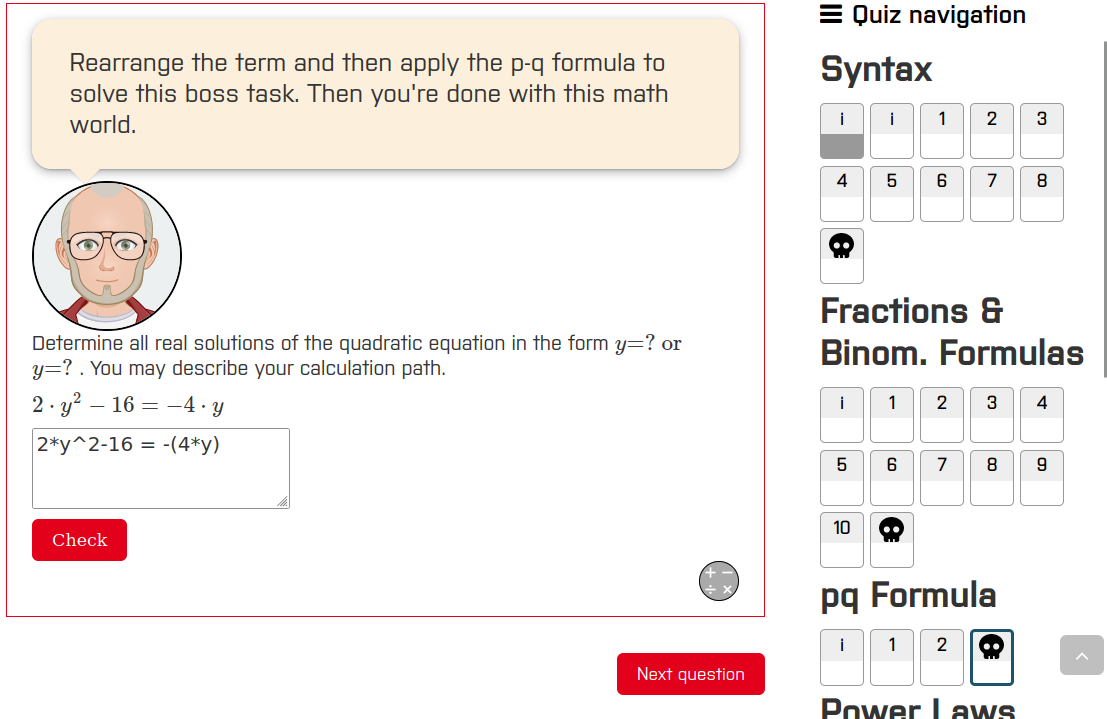
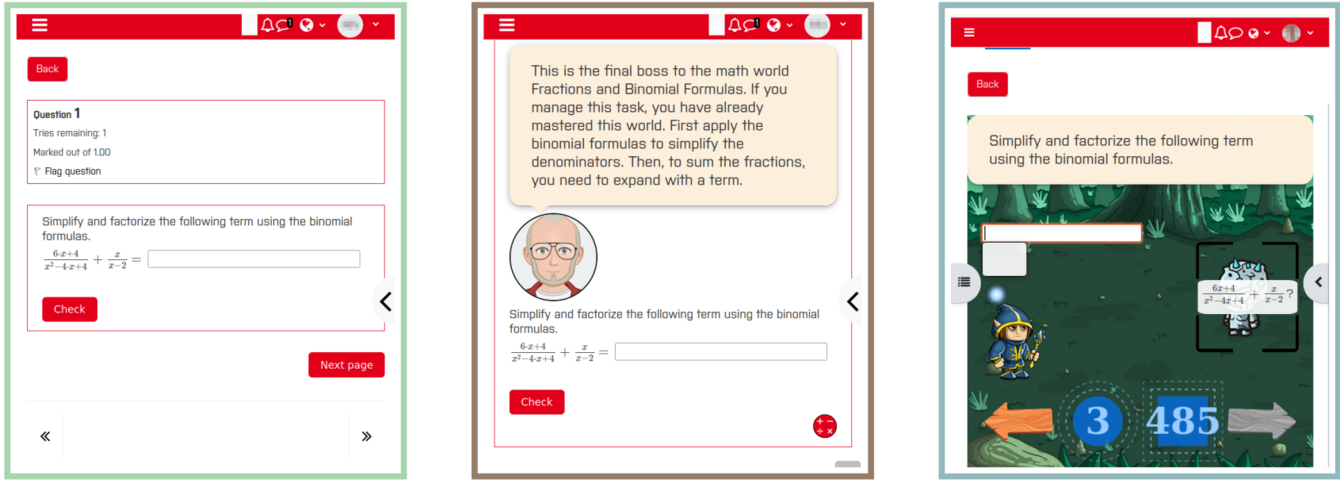
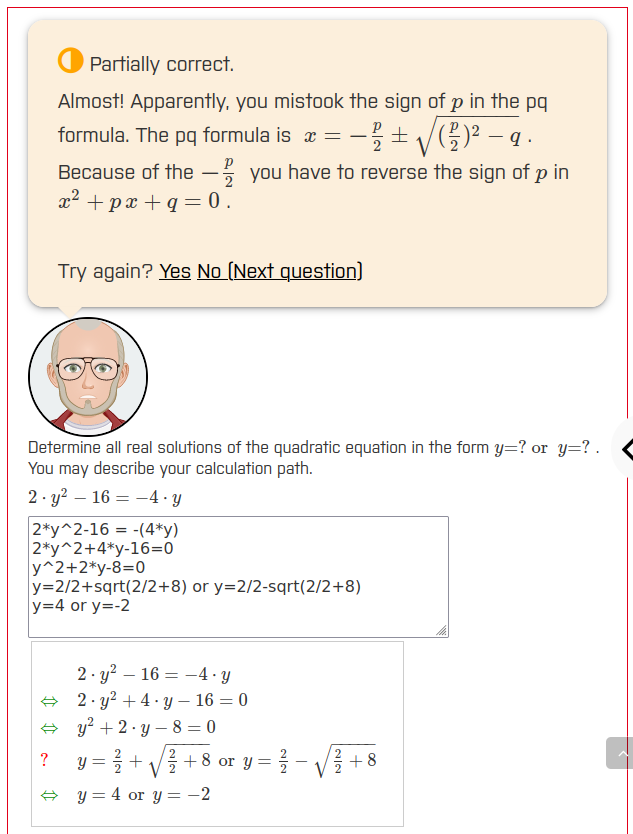
Contact
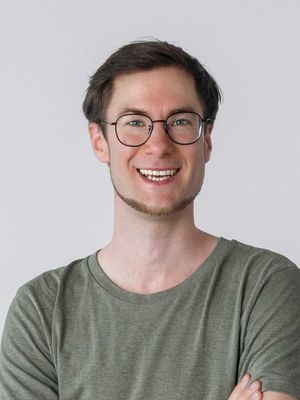