Applied Computer Science
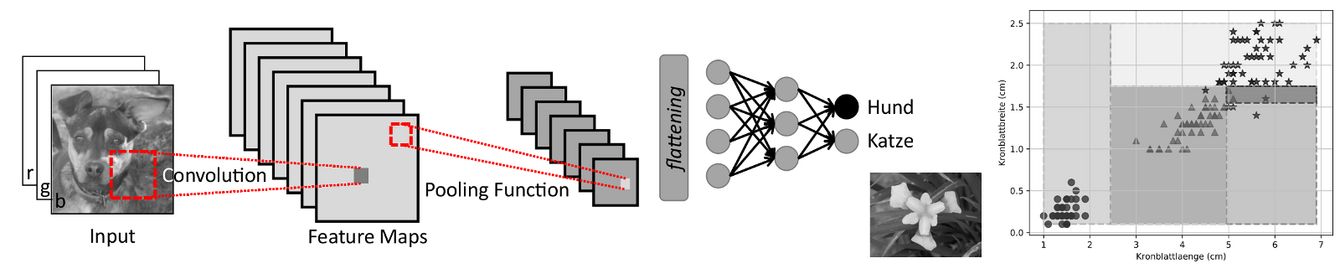
Welcome to the pages of the Applied Computer Science Group, led by Prof. Dr. Jörg Frochte. The group is part of the Department of Electrical Engineering and Computer Science and primarily operates at the Velbert/Heiligenhaus campus. Our focus in teaching and research is on artificial intelligence, machine learning, and data mining.
In the fields of machine learning and data mining, the group is well-connected both nationally and internationally. Prof. Frochte is, among other things:
- Member of the Leading Board and Founding Member of the Interdisciplinary Institute for Applied Artificial Intelligence and Data Science Ruhr (AKIS Ruhr), Bochum University of Applied Sciences. More information can be found at https://www.hochschule-bochum.de/akis/
- Member of the Graduate College NRW (PK NRW) in the Department of Computer Science and Data Science. Further details are available at https://www.pknrw.de/abteilungen/informatik-und-data-science/mitwirkende
- Associated Member of the Interdisciplinary Center for Machine Learning & Data Analytics, University of Wuppertal. More information here: https://www.izmd.uni-wuppertal.de/en/members-1/
Furthermore, numerous cooperations or contacts exist with the following universities Durham University (UK), Cardif Univesity (UK), Victoria University Wellington (NZ), Universität Leipzig (DE), Bergische Universität Wuppertal (DE), Bauhausuniversität Weimar (DE),Westfälischen Hochschule (DE), der FH Dortmund (DE)
Members of the working group
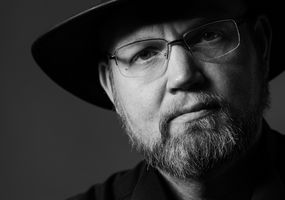
Direction | Prof. Dr. rer. nat. Jörg Frochte
Tel.: +49 2056 5848 16711
Room: 2.43 (CVH)
E-mail address: joerg.frochte@hs-bochum.de
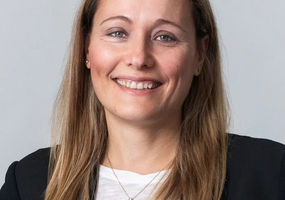
Ana Belén Martínez Torres, MEng
Tel.: +49 2056 584816741
Room: 2.40 (CVH)
E-Mail address: ana.martinez@hs-bochum.de
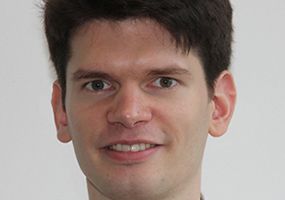
Christof Kaufmann, MEng
Tel.: +49 2056 584816743
Room: 2.40 (CVH)
E-Mail address: christof.kaufmann@hs-bochum.de
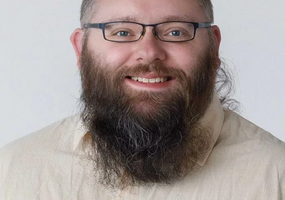
Janis Mohr, MSc
Tel.: +49 2056 584816751
Room: 2.49 (CVH)
E-Mail address: janis.mohr@hs-bochum.de
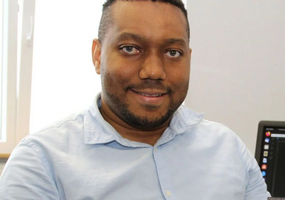
Basile Tousside, MSc
Tel.: +49 2056 584816753
Room: 2.42 (CVH)
E-Mail address: basile.tousside@hs-bochum.de
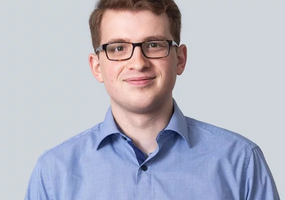
M.Eng. Stephan Sandfuchs
Tel.: +49 2056 584816746
Room: 2.42 (CVH)
E-Mail address: stephan.sandfuchs@hs-bochum.de
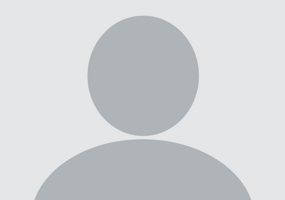
Sarah Grewe, MSc
Room: 2.42 (CVH)
E-Mail address: sarah.grewe@hs-bochum.de
Student Assistants
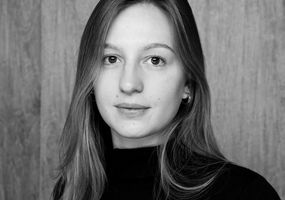
Ana Luísa S. Kelm
E-mail address: ana.schwengber-kelm@stud.hs-bochum.de

Christopher Blümer
E-Mail address: christopher.bluemer@stud.hs-bochum.de
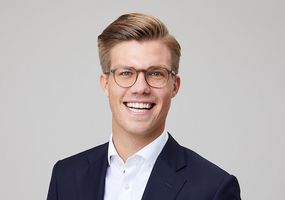
Ben Swodenk
E-mail address: ben.swodenk@stud.hs-bochum.de
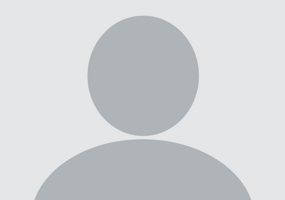
Maximilian Melchert
E-mail address: maximilian.melchert@stud.hs-bochum.de
Research
Field of Interest
The working group is active in the areas of:
- Continuous Learning: Machine learning methods and models for continuous learning.
- Machine Learning of Ill-Posed Problems: Investigation of machine learning methods to tackle mathematically unsolved problems that have no unique solutions, are sensitive to initial conditions or have no stable solution.
- Explainable AI: Techniques to improve the interpretability and transparency of AI models.
- Educational Data Mining and Learning Analytics: Improving learning outcomes and motivational factors through data-driven insights and gamification techniques.
- Data Science and Interdisciplinary Applications: Utilizing data science methods in areas such as mechatronics and robotics.
Sustainable AI and Digital Transformation: Developing energy-efficient AI technologies and supporting digital transformation in industries with a focus on environmental protection.
We are also always open to exciting new applications in the field of machine learning.
R&D Projects
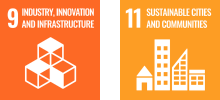
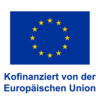
The “CoFill” project focuses on the development of AI-supported solutions to improve efficiency and sustainability in the circular economy. By using intelligent data analysis and predictive models, the aim is to optimize processes in waste management and enable a more sustainable use of resources.
- Project leader: Prof. Dr. Jörg Frochte
- Funded by: Federal Ministry of Education and Research
- Volume (in €): 375.012
- Co-op partner: Zolitron
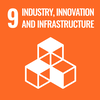
The “JetsKI” project aims to harness innovative machining processes through the use of artificial intelligence (AI). Water jet milling has enormous potential for the efficient machining of previously difficult-to-machine materials such as high-strength ceramics, but has so far been too demanding and complex for a broad range of industrial users. The application of AI methods is intended to improve process design and support the user in the computer-aided manufacturing environment in order to overcome previous limitations and achieve modern industry standards.
- Project leader: Prof. Dr. Jörg Frochte
- Funded by: NRW Innovation Promotion Agency
- Volume (in €): 1.1 million. HS Bochum's share: €536.775
- Co-op partners: HydroMill, AixPath
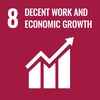
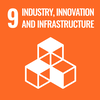
The TrAIber.NRW project, funded by the Federal Ministry for Economic Affairs and Climate Protection under the title “Action-oriented knowledge transfer in the Bergisches Land multi-pattern transformation region” (HaWiss Multitrans), supports automotive suppliers in the Bergisches Land region in coping with drive changes and climate targets.
The use of digitalization, including AI and data-driven innovations, is driving adaptations in business models and production processes. The project is developing a regional transformation strategy based on comprehensive analyses, which forms the basis for all project directions.
A particular focus is on the development of educational offerings, ranging from the adaptation of training occupations to dual study programs to promote lifelong learning and strengthen professional skills in line with SDG 4. A modular transformation toolkit also offers courses, methods and guidelines tailored to the specific needs of regional stakeholders.
The “Bergische Akademie für Transformation” will continue to provide these educational and advisory services after the end of the project funding, thus sustainably supporting SDG 9 (Industry, Innovation and Infrastructure) and SDG 13 (Climate Action) as well as SDG 4 (Quality Education) to promote comprehensive and sustainable development in the region.
- Project manager: Jörg Frochte, Prof. Dr. Clemens Faller & Prof. Dr. Markus Lemmen
- Funded by: Federal Ministry for Economic Affairs and Climate Protection
- Co-op partners: here you will find a list of the cooperation partners.
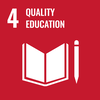
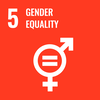
With digital mentoring, the three partner universities address several challenges that are currently influencing and shaping teaching and learning: on the one hand, there is the fact that their first semester students usually start their studies with very different prerequisites, for example with different approaches to higher education entrance qualifications and an increasing social and cultural diversity of students. In addition, the three network partners also want to take into account the increasing duration of studies and the significantly increased use of elements of digital teaching.
The digitalization of teaching in particular opens up new opportunities that digital mentoring makes use of. The “learning analytics” method used here uses the data generated by students' learning activities to better identify the needs of individual learners and provide better support for the individual learning process. To this end, the three universities use the information from various sources, such as administration and learning management systems, compile it and evaluate it in compliance with all data protection regulations. The use of Educational Data Mining is intended to help compile recommendations for the further course of study that complement the support and guidance provided by professors and service facilities such as the student advisory service.
- Project leader: Prof. Jörg Frochte
- Funded by: Foundation for Innovation in University Teaching under FBM2020-VA-219-2-05750
- Volume (in €): Bochum University of Applied Sciences' share: 948,973 euros
- Co-op partners: Dortmund University of Applied Sciences and Arts (consortium leader), Westphalian University of Applied Sciences and Arts
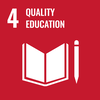
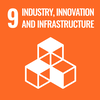
With SharKI, we are developing a shared task platform to enable teachers to implement, execute and evaluate AI-supported shared tasks in courses. The aspect of educational data mining is integrated. Primary goals at our university are robotics and control engineering.
- Project leader: Prof. Jörg Frochte
- Funded by: BMBF Call 2873
- Volume (in €): 166.545
- Co-op partners: University of Leipzig, University of Weimar
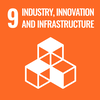
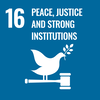
The research of this project focuses on graded learning methods. With the help of the researched approaches, we want to make progress in the following problem areas:
- Learning autonomous systems with limited resources
- Data protection & data security when learning in the cloud
- Improved handling of incomplete data sets
The project is part of the Bergisch Innovation Platform for Artificial Intelligence. This project is funded by the European Regional Development Fund (ERDF).
- Project leader: Prof. Dr. Jörg Frochte
- Funded by: EFRE (EU & NRW)
- Volume (in €): 538.310
- Co-op partners: University of Wuppertal, various industrial partners
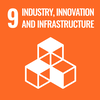
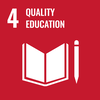
DIBS (DE: Data Mining zur Beratung von Studierenden) is an American slang expression and could probably best be translated as “First!” in German. In this sense, the aim is to implement approaches that make it possible to recognize the dangers of dropping out at an early stage. An initial study was carried out for this purpose, taking particular account of data protection and data security in learning systems and procedures.
- Project leader: Prof. Jörg Frochte
- Funded by: Ministry of Education and Science of the State of NRW
- Volume (in €): 38.850
- Co-partner: /
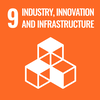
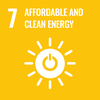
SimCloud is a project supported by the BMBF (Federal Ministry of Education and Research) for the integration of a finite element simulation into a cloud architecture. The goal is to realize an integrated workflow for a cloud-based FEM application in a prototype. Essential aspects are security and user support. For the distribution to hetrogenic structures we made intensive use of machine learning techniques. The project was funded by the BMBF.
Publications
- Maschinelles Lernen: Grundlagen und Algorithmen in Python (3. erweiterte und korrigierte Auflage)
published by the Hanser Fachbuchverlag, Nov. 2020; ISBN-13: 978-3446461444; 616 Pages - Maschinelles Lernen: Grundlagen und Algorithmen in Python (2. korrigierte Auflage)
published by the Hanser Fachbuchverlag, Jan. 2019; ISBN-13: 978-3446459960; 406 Pages - Maschinelles Lernen: Grundlagen und Algorithmen in Python (1. Auflage)
published by the Hanser Fachbuchverlag, Aug. 2018; ISBN-10: 3446446656; ISBN-13: 978-3446446656; 406 Pages - Finite-Elemente-Methode: Eine praxisbezogene Einführung mit GNU Octave/MATLAB (1. Auflage)
published by the Hanser Fachbuchverlag, Oct. 2016; ISBN-10: 3446452915; ISBN-13: 978-3446452916; 320 Pages
2024
- Bockermann, C., Frochte, J. & Schilberg, D. (2024). Angewandte Künstliche Intelligenz im Verbund verschiedener Fachbereiche. In: INFORMATIK 2024. Workshop: Aktuelle Entwicklungen und Perspektiven (an Hochschulen) im Bereich Data Science. 24-26 Semptember, Wiesbaden, Germany. DOI,PDF, BibTex
- Neugebauer, M. (2024). Lightweight Learning Analytics Dashboard for Analyzing the Impact of Feedback & Design on Learning in Mathematical E-Learning. In: Proceedings 18. Workshop Mathematik in ingenieurwissenschaftlichen Studiengängen. Pages 44-49. PDF, BibTex
- Asatyran, H., Tousside, B., Mohr, J., Neugebauer, M., Bijl, H., Spiegelberg, P., Frohn-Schauf, C. & Frochte, J. (2024). Exploring Student Expectations and Confidence in Learning Analytics. In: Proceedings of the 14th Learning Analytics and Knowledge Conference (LAK 2024). Pages 892-892, 18-22 March, Kyoto, Japan. DOI, PDF, BibTex
- Tousside, B., Frochte, J. & Meisen, T. (2024). CNNs Sparsification and Expansion for Continual Learning. In: Proceedings of the 16th International Conference on Agents and Artificial Intelligence (ICAART 2024), vol. 2, pages 110-120. 24-26 February, Rome, Italy. DOI, PDF, BibTex
- Neugebauer, M., Erlebach, R., Kaufmann, C., Mohr, J. & Frochte, J. (2024). Efficient Learning Processes by Design: Analysis of Usage Patterns in Differently Designed Digital Self-Learning Environments. In: Proceedings of the 16th International Conference on Computer Supported Education (CSEDU 2024), Vol. 2. 2-4 May, Angers, France. DOI, PDF, BibTex
- Elstner, T., Hanle, B., Loebe, F., Fröbe, M., Kolyada, N., Mohr, J., Frochte, J., Hofmann, S., Stein, B. & Potthast, M. (2024). Classification of Shared Tasks Used in Teaching. In: 29th ACM conference on Innovation and Technology in Computer Science Education (ITiCSE 2024). 8-10 July, Milan, Italy. DOI, PDF, BibTex
2023
- Mohr, J. & Frochte, J. (2023). One-Shot Identification with Different Neural Network Approaches. In: Studies in Computational Intelligence Springer book series (SCI, volume 1119). Pages 205–222. DOI, PDF, BibTex
- Neugebauer, M. & Frochte, J. (2023). Steigerung von Lernerfolg und Motivation durch gamifizierte Mathematik-Aufgaben in Lernmanagementsystemen. In: 21. Fachtagung Bildungstechnologien (DELFI). Pages 247-248. DOI, PDF,BibTex
- Mohr, J., Tousside, B., Schmidt, M., Frochte, J. (2023). Multiple Additive Neural Networks: A Novel Approach to Continuous Learning in Regression and Classification. In: 15th International Conference on Neural Computation Theory and Applications. DOI, BibTex
- Elstner, T., Loebe, F., Ajjour, Y., Akiki, C., Bondarenko, A., Fröbe, M., Gienapp, L., Kolyada, N., Mohr, J., Sandfuchs, S., Wiegmann, M., Frochte, J., Ferro, N., Hofmann, S., Stein, B., Hagen, M., & Potthast, M. (2023). Shared Tasks as Tutorials: A Methodical Approach. In: Proceedings of the AAAI Conference on Artificial Intelligence, Vol. 37 (13), 15807-15815. DOI, PDF, BibTex
- Neugebauer, M., Tousside, B. & Frochte, J. (2023). Success Factors for Mathematical e-Learning Exercises Focusing First-Year Students. In: Proceedings of the 15th International Conference on Computer Supported Education (CSEDU 2023), vol. 2, pages 306-317. ScitePress, ISBN 978-989-758-641-5. DOI, PDF, BibTex
- Asatryan, H., Gottschalk H., Lippert, M. & Rottmann, M. (2023). A Convenient Infinite Dimensional Framework for Generative Adversarial Learning. In: Electronic Journal of Statistics, vol. 17, pages 391-428. DOI, PDF, BibTex
2022
- Tousside, B., Dama, Y. & Frochte, J. (2022). Towards Explainability in Modern Educational Data Mining: A Survey. In: Proceedings of the 14th International Joint Conference on Knowledge Discovery, Knowledge Engineering and Knowledge Management. Pages 212-220. DOI, PDF, BibTex
- Tousside, B., Mohr, J., & Frochte, J. (2022). Group and Exclusive Sparse Regularization-based Continual Learning of CNNs. In: Proceedings of the Canadian Conference on Artificial Intelligence. DOI, PDF, BibTex
- Quetscher, F., Kaufmann, C. & Frochte, J. (2022). Investigation of Capsule Networks Regarding their Potential of Explainability and Image Rankings. In: Ana Paula Rocha, Luc Steels und H. Jaap van den Herik (Hg.): Proceedings of the 14th International Conference on Agents and Artificial Intelligence, ICAART 2022, vol. 3, pages 343 - 351. Online Streaming, February 3-5, ScitePress. DOI, PDF, BibTex
- Sandfuchs, S., Schmidt, M. & Frochte, J. (2022). Novel Approaches for Periodic Depth Enhancement in Visual SLAM. In: Andreas Müller und Mathias Brandstötter (Hg.): Advances in Service and Industrial Robotics. Cham: Springer International Publishing, pages 436–443. DOI, PDF, BibTex
- Tousside, B., Friedrichsen, L. & Frochte, J. (2022). Towards Robust Continual Learning using an Enhanced Tree-CNN. In: Ana Paula Rocha, Luc Steels und H. Jaap van den Herik (Hg.): Proceedings of the 14th International Conference on Agents and Artificial Intelligence, ICAART 2022, volume 3. Online Streaming, February 3-5. DOI, PDF, BibTex
2021
- Mohr, J., Tousside B., Schmidt, M. & Frochte, J. (Hg.) (2021a). Explainability and Continuous Learning with Capsule Networks. In Proceedings of the 13th International Joint Conference on Knowledge Discovery, Knowledge Engineering and Knowledge Management (IC3K 2021), volume 1: KDIR, pages 264-273. ISBN: 978-989-758-533-3; ISSN: 2184-3228; INSTICC: ScitePress. DOI, PDF, BibTex
- Mohr, J., Breidenbach, F. & Frochte, J. (2021). An Approach to One-shot Identification with Neural Networks. In: Thomas Bäck, Christian Wagner, Jonathan M. Garibaldi, H. K. Lam, Marie Cottrell, Juan Julián Merelo und Kevin Warwick (Hg.): Proceedings of the 13th International Joint Conference on Computational Intelligence, IJCCI 2021, pages 344–351. Online Streaming, October 25-27, ScitePress. DOI, PDF, BibTex
2020
- Frochte, J. & Marsland, S. (2020). Regression learning on patches. In: 2020 IEEE Symposium Series on Computational Intelligence (SSCI), pages 1786–1793. DOI, PDF, BibTex
- Frochte, J., Lemmen, M. & Schmidt, M. (2020). Concerning the Integration of Machine Learning Content in Mechatronics Curricula. In: Revolutionizing Education in the Age of AI and Machine Learning: IGI Global, pages 75–96. DOI, PDF, BibTex
- Tousside, B., Mohr, J., Schmidt, M. & Frochte, J. (2020). A Learning Approach for Optimizing Robot Behavior Selection Algorithm. In: Chan, C. S., Liu, H., Zhu, X., Lim, C. H., Liu, X., Liu, L. & Goh, K. M. (Hg.): Intelligent Robotics and Applications - 13th International Conference, ICIRA 2020, Kuala Lumpur, Malaysia, November 5-7, 2020 Springer (Lecture Notes in Computer Science), vol. 12595, pages 171–183. DOI, PDF, BibTex
2019
- Börzel, S. & Frochte, J. (2019). Case Study on Model-based Application of Machine Learning using Small CAD Databases for Cost Estimation. Proceedings of the11th International Joint Conference on Knowledge Discovery, Knowledge Engineering and Knowledge Management, pages 258–265. DOI, PDF, BibTex
- Frochte, J. & Marsland, S. (2019). A Learning Approach for Ill-Posed Optimisation Problems. In: Data Mining, pages 16–27. Springer Singapore. DOI, PDF, BibTex
2018
2016
- Bernst, I., Kaufmann, C. & Frochte, J. (2016). On learning assistance systems for numerical simulation. In: International Journal on Computer Science and InformationSystems, 11 (1), pages 115–133. PDF, BibTex
- Frochte, J. & Bernst, I. (2016). Success Prediction System for Student Counseling Using Data Mining. In: Proceedings of the 8th International Conference on Knowledge Discovery and Information Retrieval, pages 181–189. DOI, PDF, BibTex
- Kaufmann, C. & Frochte, J. (2016). A Case Study on FMU as Co-Simulation Exchange Format for FEM Models. In: Proceedings of the 13th International Conference on Applied Computing 2016 (Mannheim, Co-Organized by the University of Mannheim), vol. 12595. October 2016. ISBN 978-989-8533-56-2. PDF, BibTex
2015
- Bernst, I., Kaufmann, C. & Frochte, J. (2015). Learning Load Balancing for Simulati-on in Heterogeneous Systems. In: H. Weghorn (Hrsg.), Proceedings of the 12th International Conference on Applied Computing 2015, pages 121–128. DOI, PDF, BibTex
- Bouillon, P., Frochte, J. & Lemmen, M. (2015). Influence of plant model variants for the automatic optimisation of control parameters. In: 16th International Conference on Research and Education in Mechatronics (REM), pages 80–87. DOI, BibTex, PDF
- Bouilon, P. & Frochte, J. (2015). Simulation- and Web-Based E-Learning in Engineering - Open Source Architecture and Didactic Issues -. In: Proceedings of the IEEE 16th International Conference on Research and Education in Mechatronics, pages 127-134. DOI, PDF, BibTex
2014
- Bernst, I., Bouillon, P., Frochte, J. & Kaufmann, C. (2014). An approach for load balancing for simulation in heterogeneous distributed systems using simulation datamining. In H. Weghorn (Hrsg.), Proceedings of the 11th International Conference on Applied Computing 2014, pages 254–238. DOI, PDF, BibTex
- Frochte, J., Kaufmann, C. & Bouillon, P. (2014). An approach for secure cloud computing for FEM simulation. In H. Weghorn (Hrsg.), Proceedings of the 11th International Conference on Applied Computing 2014, Spages 234–238. DOI, PDF, BibTex
2013
- Burrows, S., Frochte, J., Völske, M., Torres, A. B. M. & Stein, B. (2013). Learning Overlap Optimization for Domain Decomposition Methods. In J. Pei, V. S. Tseng,L. Cao, H. Motoda & G. Xu (Hrsg.), Proceedings of the Seventeenth Pacific-Asia Conference on Knowledge Discovery and Data Mining, pages 438–449. Springer. DOI, BibTex, PDF
2011
Teaching
Members of the working group supervise the following courses at the Velbert/Heiligenhaus campus of Bochum University of Applied Sciences.
Bachelor programme
Password and login for the documents will be announced in the course.
- Analysis 2 (documents, aids for the exam etc.)
- Data analysis and data visualization (documents etc.)
- Machine learning (documents etc.)
- Artificial Intelligence
“Machine Learning & Data Mining” can also be taken as an elective module in the Computer Science degree programmes (Bochum campus) at CVH.
Master's programme
The following courses are offered as part of the Master's degree programs in Mechatronics and Product Development as well as Computer Engineering at CVH:
- Applied Artificial Intelligence & Machine Learning ( documents etc.)
- Seminar on current topics in AI and data science (as part of the AKIS seminar)
** The teaching langague for all seminars mentioned above is German
- 2024 – M. Korostovskiy: Bachelorarbeit mit dem Thema “Analysis and Comparison of Machine-Learning Algorithms for Inventory and Sales Forecasting”
- 2024 – K. Wittke: Bachelorarbeit mit dem Thema “Comparative Analysis of Reinforcement Learning Algorithms with Discrete Action Spaces”
- 2023 - A. J. Fanyim Kamga: Bachelor thesis with the topic “Measuring Customer Satisfaction and Identifying Potential Improvement Areas in a Convenience Store using Customers Reviews & NLP-Techniques.”
- 2023 - M. Wallitschek: Bachelor thesis with the topic “Generation of descriptions of individual image elements by machine learning to increase the explainability of a classification”
- 2022 - T. Überlackner: Bachelor thesis with the topic “Development of a predictive maintenance system based on a deep learning model”
- 2022 - M. Born: Bachelor thesis with the topic “Analysis of the suitability of piezoelectric ceramic capacitors in frequency-variable resonance inverters for controlling BLDC motors in electrified drives”
- 2022 - M. Heimbach: Master's thesis with the topic “Performance comparison of different reinforcement learning methods on the limited computing resources of a crawling robot”
- 2021 - F. Quetscher: Master's thesis with the topic “Investigation of Capsule Networks regarding the Explainability of Search Engine Rankings”
- 2021 - S. Meier: Master's thesis with the topic “Analysis and comparison of methods in predictive maintenance”
- 2020 - L. Friedrichsen: Master's thesis with the topic “Impact of modularization on learning behavior and detection rate of Convolutional Neural Networks”
- 2020 - F. Breidenbach: Bachelor thesis with the topic “Object recognition by machine learning using surface textures - approach based on Convolutional Neural Network”
- 2019 - H. Richter: Master's thesis with the topic “Possible applications of convolutional neural networks for the classification of bird calls”
- 2019 - L. Jakob: Master's thesis on “Transfer learning for hand gesture recognition with low-resolution image data”
- 2018 - H. Richter: Bachelor thesis with the topic “Information extraction from ID documents using deep neural networks”
- 2017 - P. Bouillon: Master's thesis with the topic “Simulation-Based Pretraining for a Multilevel Reinforcement Learning on Mobile Robots”
- 2017 - Tobias S. Fischer: Bachelor thesis with the topic “Nonintrusive Load Monitoring - Recognition of Finite State Machines using Sequential Pattern Mining”
- 2016 - J. Beran: Master thesis with the topic: “Comparison of different approaches for learning agents in strategic business games”
- 2015 - T. E. Preuß: Bachelor thesis with the topic: “Training of a vacuum robot in a virtual environment”
- 2015 - O. P. Müller: Master's thesis with the topic: “Classification of hand gestures in three dimensions using machine learning”
- 2015 - J. Dambacher: external bachelor thesis
- 2014 - P. Bouillon: Bachelor's thesis with the topic: “Training a humanoid robot using machine learning”
- 2014 - D. Cziesla: Bachelor's thesis with the topic: “3D visualization of technical data in web applications”
Interested in writing a thesis in applied computer science?
If you are a student at Bochum University of Applied Sciences and are thinking about writing a Bachelor's or Master's thesis with us, you can find out more about the focus of the working group in the sections Projects and Theses, Research and Development, and Publications. In our experience, the following generally applies: If you come up with your own idea or we find a topic together that really interests you, the results will generally be good. So if your topic does not appear in the subject areas on these pages, don't let this put you off at first, but simply present your idea once.
As far as the form is concerned, the same instructions apply as below for term papers. If you are unsure, it is best to address the open questions directly at the beginning of the paper.
The working group regularly offers the option of carrying out cooperative, software and development projects in the context of its own thematic focus. Suggested topics are regularly posted in the showcases. Of course, you are also welcome to come up with your own ideas.
Computer science students from Bochum with an interest in machine learning or applied AI are of course also welcome for their software projects etc.
Here you will find a rough guide to writing a thesis or term paper, as a pdf and as a complete LaTeX template (zip) (both in German).
Please consider this example as a guide only. It should give you an impression of how a paper is typically structured and which classic mistakes (see e.g. note on handling sources and citations at the bottom of the page) should be avoided. Even the basics do not always have to be called basics, but can be modified as a heading for the paper.
Of course, you do not have to use LaTeX. If you want to use Word or LibreOffice, you can simply follow the pdf above, and if you want to use LaTeX under Windows, we recommend MiKTeX.
Please note that form and citation technique are important aspects of such papers and are also reflected in the grade. Take these tips to heart.