Green and Trustworthy AI
Green AI
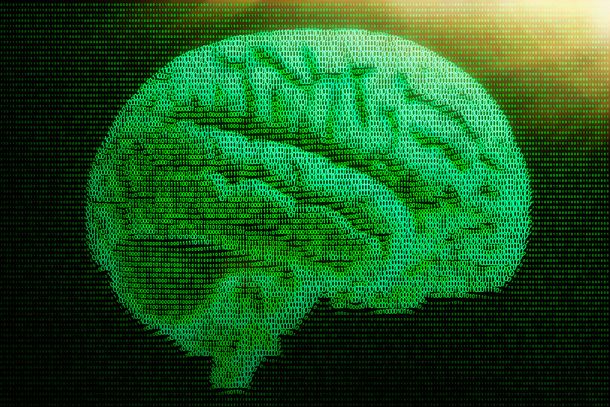
In recent years, artificial intelligence has been on everyone's lips. Autonomous driving, image recognition and robotics are just a few of the application areas in which the term Artificial Intelligence or Machine Learning is heard more and more often. AI (artificial intelligence) is used to recognize street signs and faces, learn motion sequences in complex situations or strategies in the game GO. In current research in these areas, the primary focus is on "accuracy". The quality of an AI is very much measured by how well it can distinguish between dogs and cats, for example. Currently, good accuracy is often achieved by expending large amounts of computing power along with appropriate data sets. This brings two serious problems. It is becoming increasingly difficult to participate in development with common resources such as a single workstation, which means that research is concentrated on a few big players with large budgets. In addition, large amounts of energy are used and CO2 is generated to train "state-of-the-art" artificial intelligence. Information and communication technology now accounts for 3.5% of global CO2 emissions and even training a single model can generate 1t or more of CO2. This trend, also called Red AI, is contrasted with Green AI. Green AI also means giving greater consideration to factors such as computation time and efficiency when evaluating artificial intelligence. This promotes methods that take sustainability aspects into account and act in an environmentally friendly manner. Artificial intelligence that can be trained and used by SMEs or smaller research groups, even without large data centers, could be the result. Green AI is a push to consider efficiency as a factor in the evaluation of machine learning methods and thus to promote approaches that are not exclusively optimized for accuracy, but also weigh resource consumption and the sustainable use of a model.
Trustworthy AI
From a technological perspective, artificial intelligence has made tremendous progress in recent years - increasingly complex data and applications can be predicted and supported with models. This enables ever more advanced automated decision making and highly optimized decisions in many areas. The temptation is to put more and more decisions in the hands of data-based models.
However, development on the non-AI side - i.e., primarily in the actual applications - is much slower, and the outsourcing of decisions raises a number of questions that our society needs to address from both social and economic perspectives:
- Can we understand the decisions made by AI models? (Transparency)
- Do the AI results lead to better outcomes overall? (Context)
- Does the basis (data) of AI decisions correspond to actual circumstances? (Data quality)
- Are the AI decisions fair? (Impartiality)
- Who takes responsibility of AI decisions? (Legal certainty)
The further development of AI is now also about establishing not only the technical methods, but also the transparency and acceptance of AI. The aforementioned aspects are affected along the entire processing chain of AI processes (data recording, data pre-processing, model building, application). Therefore, the development of Trustworthy AI goes hand in hand with the further development of technological models, but also with the establishment of structural and organizational processes.