Educational Data Mining
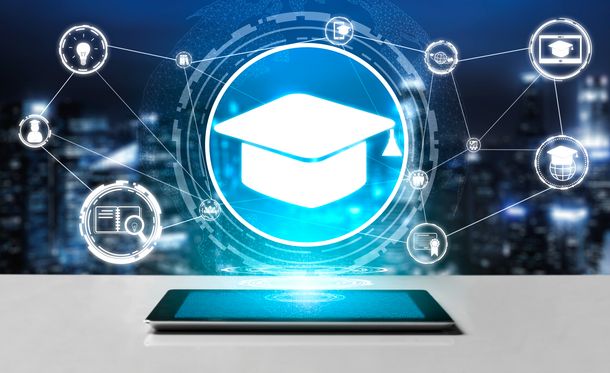
Educational Data Mining is - simplified spoken - data mining on data, which are created or collected in the educational sector. Emerging means data that simply accrue without a specific goal in research or even to improve programs. This is typically administrative data that accumulates in the databases of schools and colleges; for example, the data that has been collected for many years to see where hurdles exist in a course of study or what the conditions are that lead to a school or college biography being successful. In this field, the focus is always on the database, classical statistics and techniques from the field of machine learning. Educational data mining can take place on this level of entire school and study histories or on the level of individual courses or techniques. In these cases, the goal is to use educational data from the use of interactive learning environments by e.g. students from computer-based collaborative learning platforms. The goal is always to better understand learners and the environments in which they learn; sometimes in the process of designing software to help adaptively support learning processes. Someone doing data mining on educational data is not automatically a didactician or educator in this process. For a few questions, which are more reminiscent of suggestion systems of sales platforms, here only for tasks etc., this is not always necessary. But the full potential of this application can only be realized when working in interdisciplinary teams, so that didacticians and educators accompany interpretations from the patterns obtained by statistics and machine learning and classify results. In addition, educational data is very sensitive data. We are talking about people who are being looked over their shoulders at something they do not yet know how to do; voluntariness here being an aspect that can be subsumed under data protection to some extent, precisely because there has to be a declaration of consent for the data processing. Another point, however, is explainability as a partial aspect of Trustworthy AI. In order to avoid perpetuating discrimination that may have manifested itself in past learning biographies, we must always be able to understand and question the "Why" when making assessments of AI systems. The potential of this application of machine learning is very large to improve educational equity and quality. At the same time, it is one of the areas where the application of data-driven innovation comes with a special responsibility and where we need to capitalize on the idea of Trustworthy AI.